New paper in Neural Networks - a deep neural network learns to produce new words
The paper proposes two neural network architectures (fiwGAN and ciwGAN) to model unsupervised learning of spoken words from raw audio and proposes a new latent space structure that can model featural learning and allows for a very low-dimension vector representation of lexical items.
The proposed network generates innovative outputs that are linguistically interpretable and highly informative. fiwGAN network trained on suit and dark outputs innovative start, even though it never saw start or even a [st] sequence in the training data (audio sample below).
We also argue that setting latent featural codes to values well beyond training range results in almost categorical generation of prototypical lexical items and reveals underlying values of each latent code.
The proposed architecture allows testing of any property of the data and probing the learned representation behind each latent code. Lexical learning emerges even when trained on the entire TIMIT database.
FiwGAN allows very low dimension vector representation (13 binary latent variables) of lexical items when trained on TIMIT (~6k lexical items, 55k tokens). Approx. 40% of tested codes have a distinct and easily identifiable lexical item (spectrograms below).
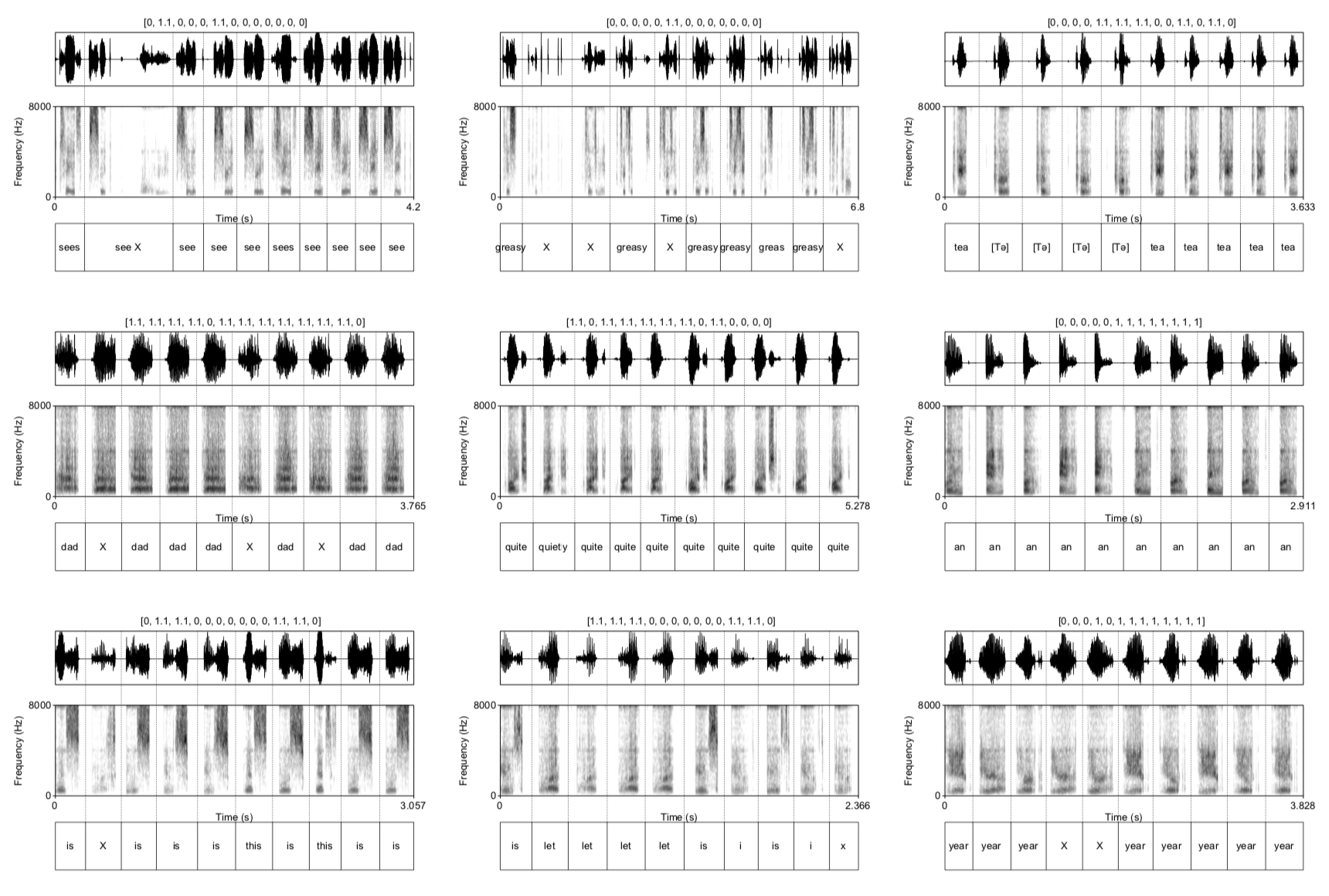