A new preprint on comparing artificial and biological neural computation
Human brain and deep learning models show some crucial similarities in how sounds of speech are processed.
Preprint with Alan Zhou & Christina Zhao: bioRxiv
We propose a framework for paralleling intermediate layers in unsupervised deep neural networks and complex Auditory Brainstem Response (ABR).
The proposed method allows a direct and interpretable comparison that does not focus on correlations, but instead on a direct comparison of acoustic properties between the signals (no transformations needed).
We introduce GANs to the brain-ANN comparison paradigm, which allows testing of both production and perception principles (decoding & encoding) in a fully unsupervised manner.
We argue that peak latency to VOT in the brain stem differs in similar and interpretable ways between English and Spanish speakers in the ABR experiment, and in intermediate convolutional layers between English-trained and Spanish-trained computational models.
Any other acoustic property that results in a phonological contrast can be tested using the proposed methods.
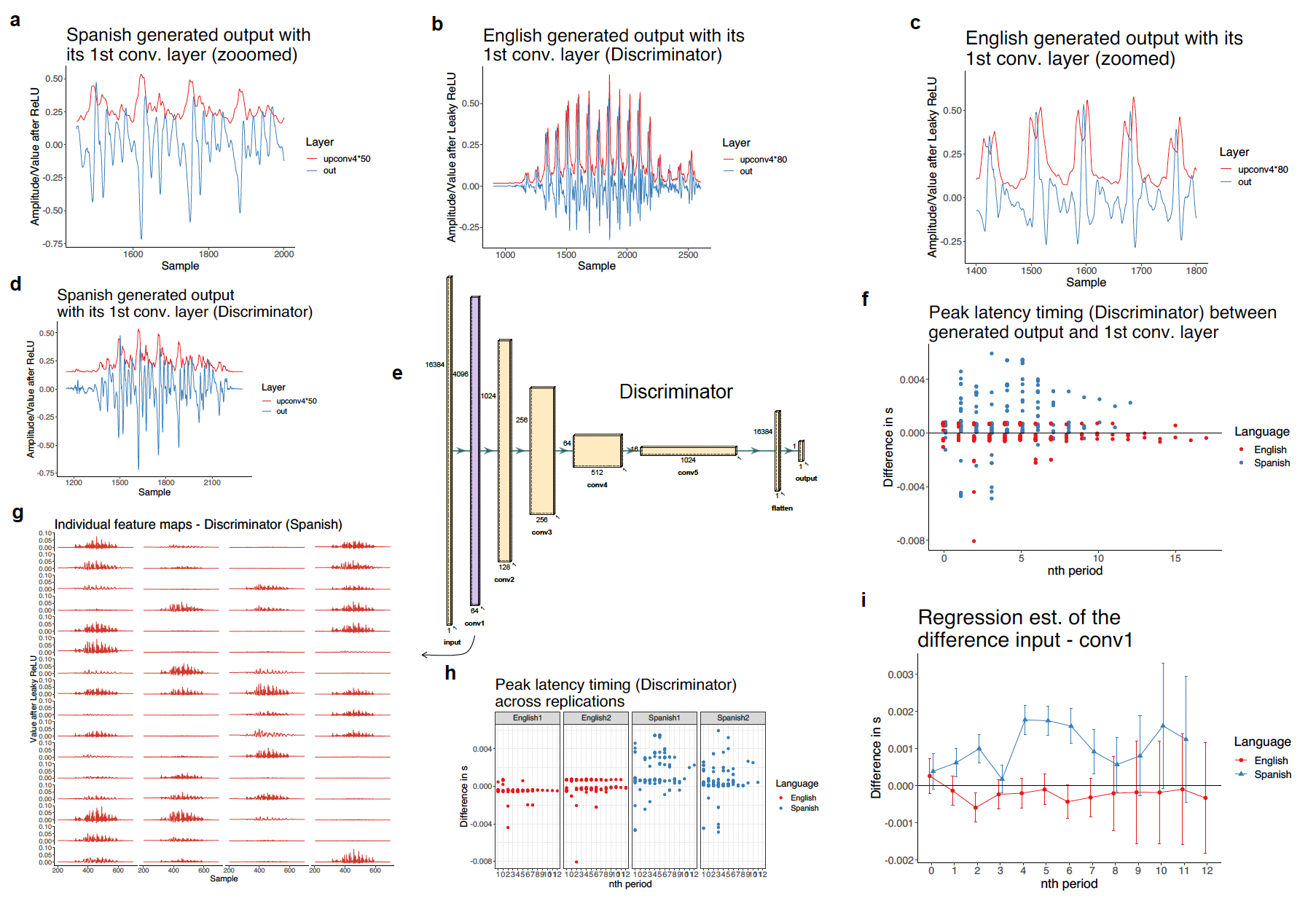