Can deep convolutional network learn identity-based patterns? A new preprint is on arXiv
We train a ciwGAN model on reduplication in speech (a syllable [pa] gets copied, e.g. [para] -> [pa-para]). The network learns to encode an identity-based pattern in its latent space & learns to apply it to unobserved data.
Pre-printarXiv.
For example, manipulating only the latent codes, we can turn an unreduplicated output [piru] into a reduplicated one [pipiru]. Waveform below shows a gradual transition from [piru] to [pipiru] with interpolation of latent codes.
Reduplication (copying) is extended to unobserved data. [s]-initial words were never reduplicated in training. With a proposed technique, we can force both [s] and reduplication. The network outputs [sisiji], very similar to human outputs that were withheld from training (below).
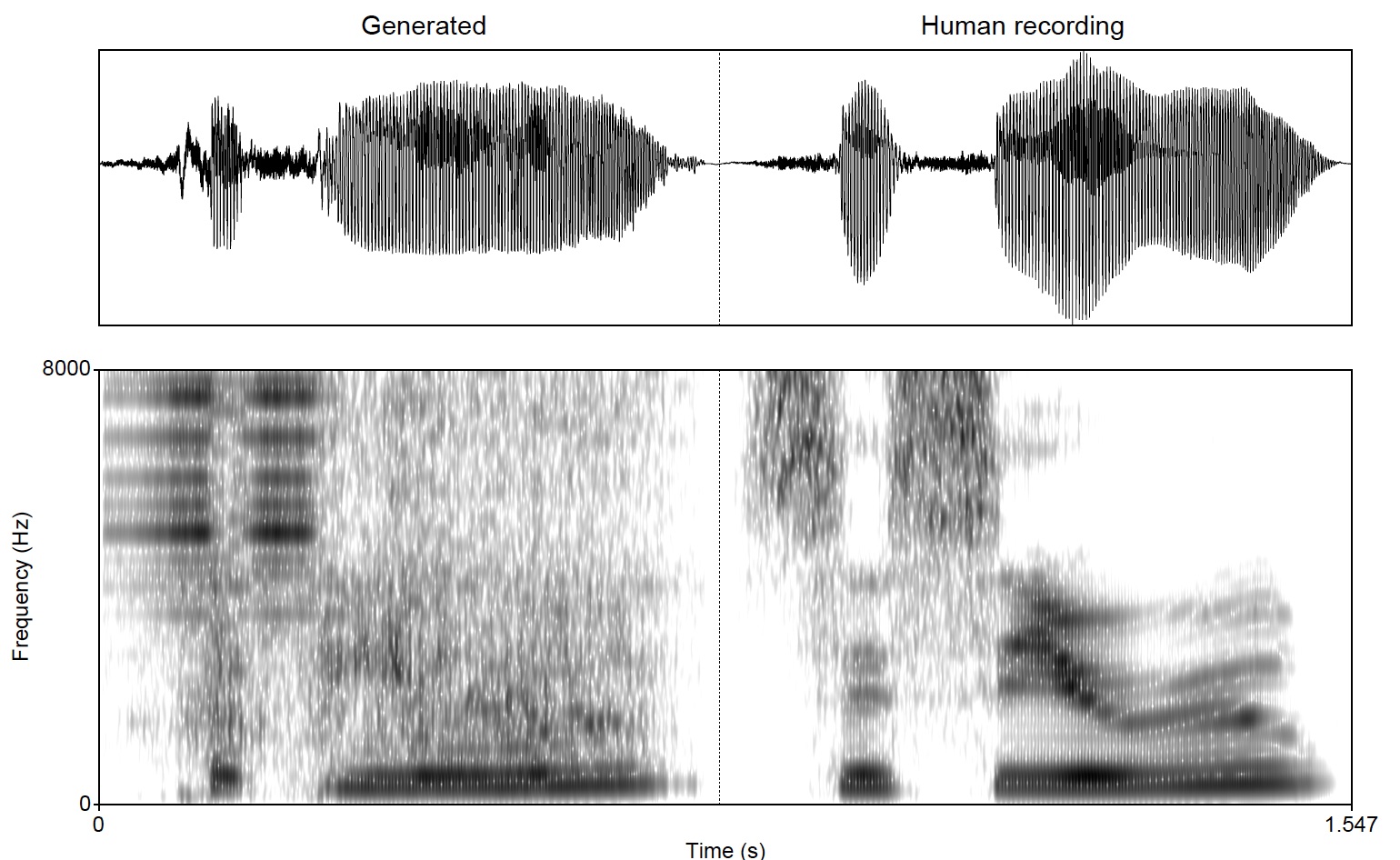
Generated outputs and human recordings.